RADARS
AI-based anomaly detection for 5G/6G networks
- You are here
- Company
- Research projects
- RADARS
Vulnerable network generation 5G/6G
5G and 6G networks are complex and far-reaching, making them vulnerable to cyberattacks - both on the Radio Access Network (RAN) and on the core network. These attacks can lead to service disruptions, data breaches or vulnerabilities in critical infrastructures. A robust security foundation - especially for the configuration of small cells and IoT sensor technology in unprotected environments - is crucial for protection.
Project RADARS: Developing a robust security framework
In the RADARS research project, we are working with Chemnitz University of Technology on the development of a robust security framework for 5G/6G networks, including the base stations and clients used there. The consideration of Open RAN (O-RAN) components is particularly important here. This is because the application in the “Internet of Things” and “Industry 4.0” sectors and the complexity of the “modular principle” of open solutions result in completely different requirements in terms of scalability, control and management levels, traffic flows and their attack vectors or Indicators of Compromise (IoCs).
Main research areas
- Automation of the optimal configuration of Open RAN components in accordance with manufacturer and BSI guidelines
- Analysis of asset detection and preventive vulnerability scans
- Development of methods for autonomous anomaly detection in compliance with data protection guidelines
Expansion of the AI-supported security system for 5G/6G networks
Our approach is to extend an existing AI-powered intrusion detection and response system to 5G and 6G communication technologies. In a demo environment, we will integrate this system into the operational environment to efficiently correlate security incidents and enable consistent tracking. An open source-based Security Information and Event Management (SIEM) will be used for this purpose.
Federated learning: solution for data protection
In contrast to conventional machine learning-based intrusion detection systems (ML-IDS), which are questionable from a data protection perspective due to their centralized approach, this project also considers federated learning (FL)-based approaches as a decentralized alternative solution. This lays the technical foundation for the operation of a Security Operations Center (SOC) including a BSI reporting office for 5G/6G infrastructures via an open source security kit, also from a data protection perspective.
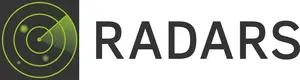
RADARS is a BSI initiative
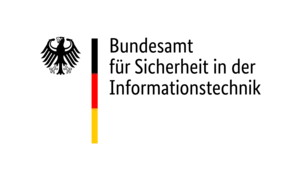